File:CRTC QCNN NP1.png
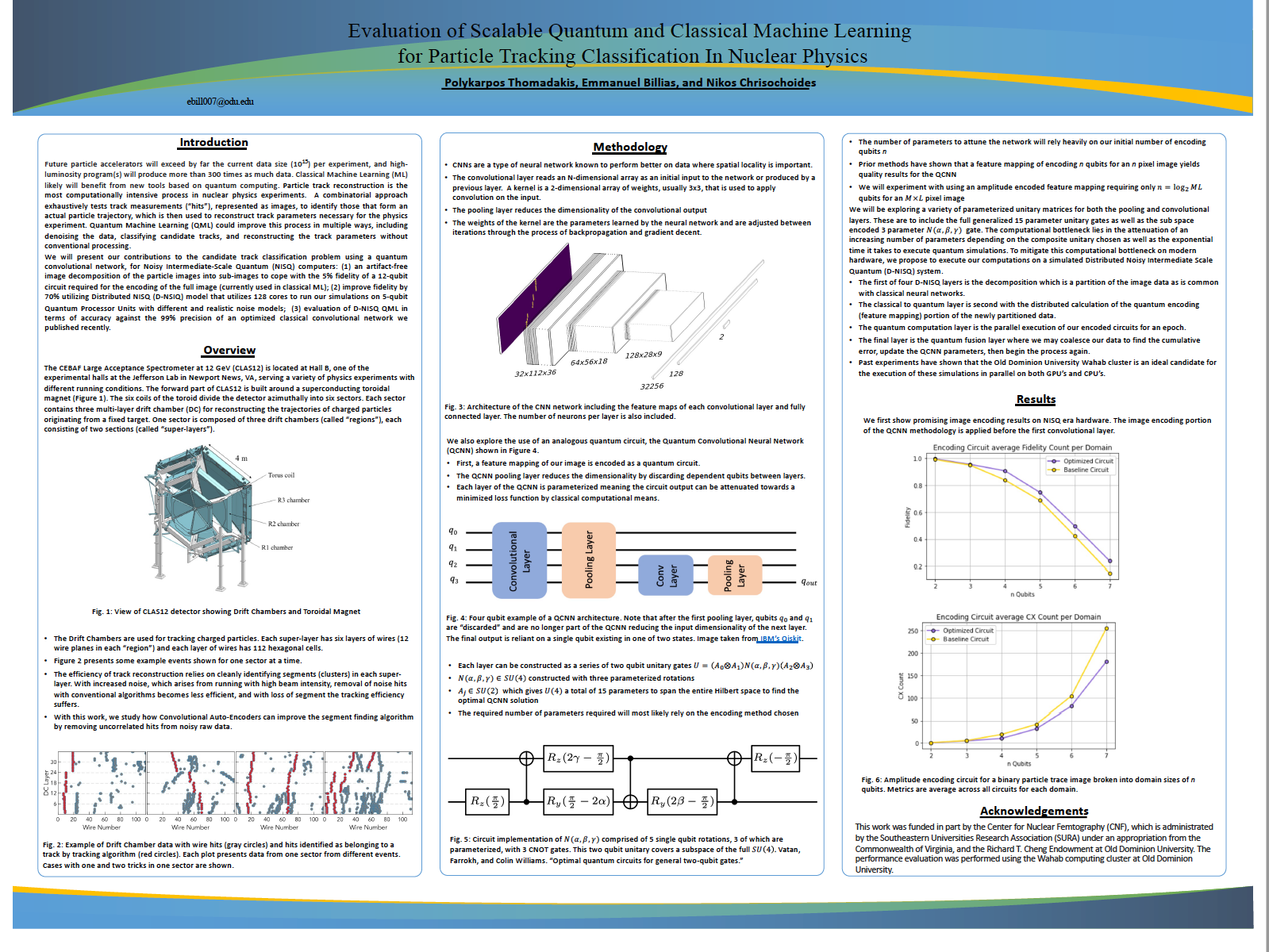
Future particle accelerators will exceed by far the current data size (1015) per experiment, and high-luminosity program(s) will produce more than 300 times as much data. Classical Machine Learning (ML) likely will benefit from new tools based on quantum computing. Particle track reconstruction is the most computationally intensive process in nuclear physics experiments. A combinatorial approach exhaustively tests track measurements (“hits”), represented as images, to identify those that form an actual particle trajectory, which is then used to reconstruct track parameters necessary for the physics experiment. Quantum Machine Learning (QML) could improve this process in multiple ways, including denoising the data, classifying candidate tracks, and reconstructing the track parameters without conventional processing. We will present our contributions to the candidate track classification problem using a quantum convolutional network, for Noisy Intermediate-Scale Quantum (NISQ) computers: (1) an artifact-free image decomposition of the particle images into sub-images to cope with the 5% fidelity of a 12-qubit circuit required for the encoding of the full image (currently used in classical ML); (2) improve fidelity by 70% utilizing Distributed NISQ (D-NSIQ) model that utilizes 128 cores to run our simulations on 5-qubit Quantum Processor Units with different and realistic noise models; (3) evaluation of D-NISQ QML in terms of accuracy against the 99% precision of an optimized classical convolutional network we published recently.
File history
Click on a date/time to view the file as it appeared at that time.
Date/Time | Thumbnail | Dimensions | User | Comment | |
---|---|---|---|---|---|
current | 06:47, 10 February 2023 | ![]() | 1,599 × 1,200 (653 KB) | Nikos (talk | contribs) | Future particle accelerators will exceed by far the current data size (1015) per experiment, and high-luminosity program(s) will produce more than 300 times as much data. Classical Machine Learning (ML) likely will benefit from new tools based on quant... |
- You cannot overwrite this file.
File usage
The following page links to this file: